Spring/Summer 2019 Featured Research Profile
“A man is worked upon by what he works on. He may carve out his circumstances, but his circumstances will carve him out as well.”
– Frederick Douglass.
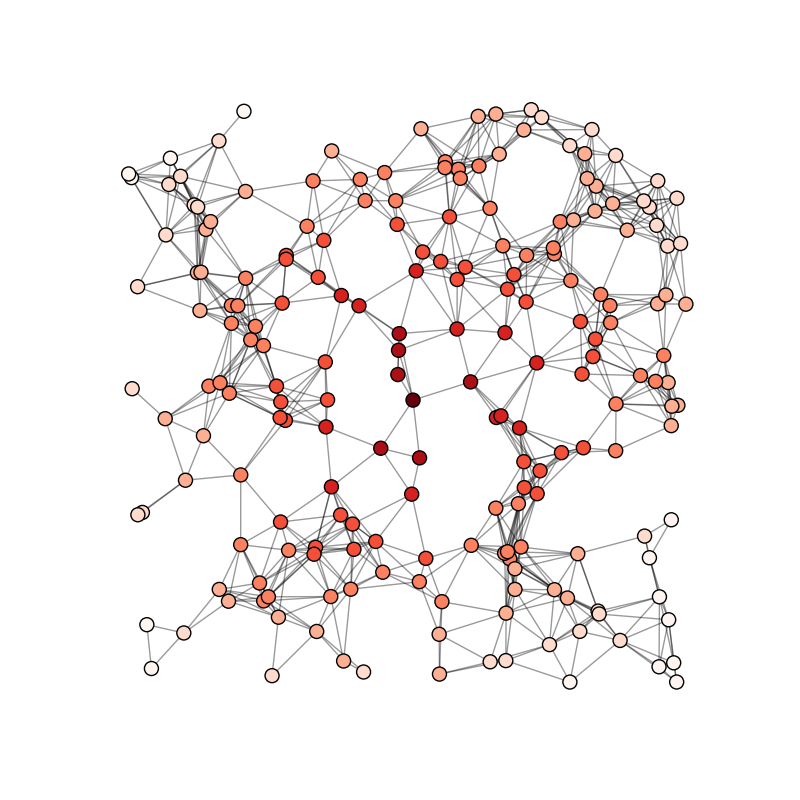
GCER Fellow Alexandre Poirier‘s and Matthew Masten’s study of treatment effects carves out the circumstances under which circumstances carve out economic behavior.
Economists handling data need to make assumptions to draw causal conclusions. For example, to evaluate the effect of participating in a job training program on unemployment duration, one has to take a stand on how program participation is related to the participants and nonparticipants’ characteristics. These questions can sometimes be answered with randomized experiments, but more often economists must work with observational data. In this case, researchers often make a “quasi-randomization” assumption that allows us to analyze the data as if a certain randomized experiment was actually performed. Since an experiment was not actually performed, it is important to understand what conclusions we can draw when assumptions like quasi-randomization fail.
In two recent papers entitled “Identification of Treatment Effects under Conditional Partial Independence” and “Inference in Breakdown Frontiers” (both co-authored with Matthew Masten at Duke University), Georgetown economist and GCER Fellow Alexandre Poirier propose a framework to address the question: how sensitive are empirical results to assumptions like quasi-randomization?
Poirier and Masten first define a measure of “distance from independence” which quantifies departures from quasi-randomization. Under independence, the average effect of a treatment (such as participation in a job training program) can be recovered from the data, while under partial independence one can only recover a range of values for this average treatment effect. This range increases as one departs further from quasi-randomization. This range can be used by economists to determine the sensitivity of their results to quasi-randomization failures.
Second, they also determine the “breakdown” for different conclusions that are typically made. For example, economists are often interested in finding out whether at least half of economic agents would benefit from a treatment. Using Masten and Poirier’s framework, economists can determine which set of assumptions guarantee that at least half benefit from a treatment, and which set of assumptions does not yield that conclusion. This allows researchers to assess the sensitivity of conclusions with respect to one or more assumptions that cannot be verified in the data. In particular, if a large number of different assumptions guarantee that at least half benefit, then this result can be deemed robust. Meanwhile, if very few assumptions lead to this conclusion, it will be deemed fragile. Their framework also allows researchers to examine the tradeoffs between imposing and relaxing different assumptions.
Finally, Poirier and Masten apply their method to a previous study by Blattman and Annan (Rev Econ and Stat 2010) on the effects of child soldiering on economic outcomes. The techniques of Poirier and Masten determine which conclusions in the Blattman and Annon study are more or less robust to violations of the quasi-experimental assumptions.